Customer Segmentation and Churn Modelling for Effective E-commerce
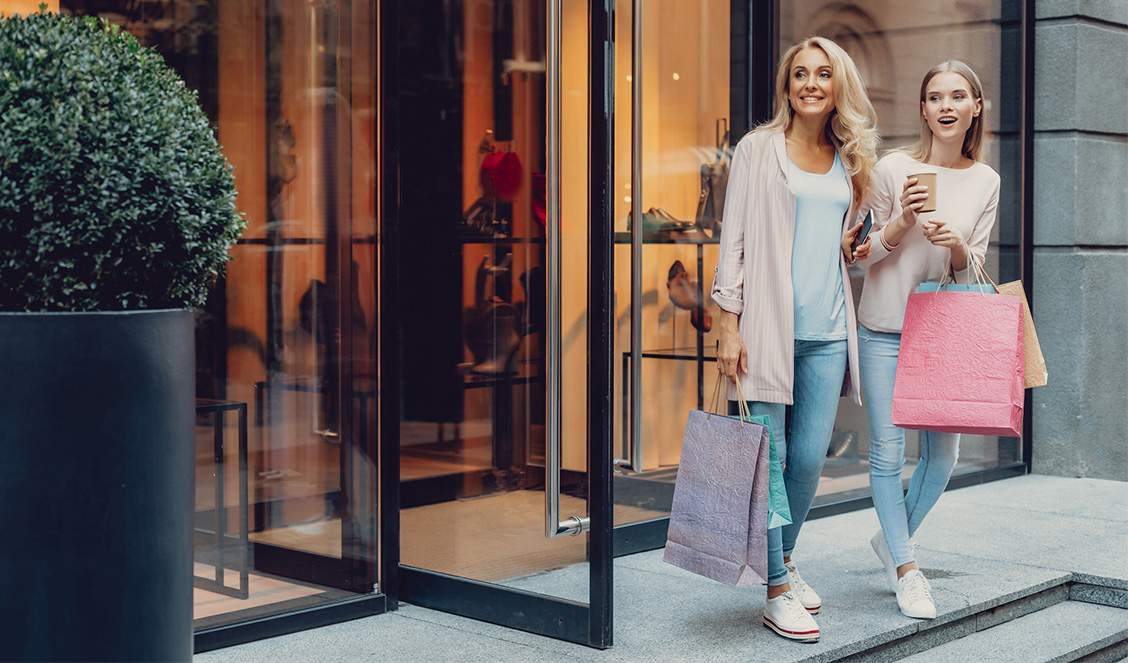
What is customer churn?
Customer churn is a number, or percentage, of customers that stop using a company’s product, or service, within a certain period of time e.g., one year.
Customer churn is an important metric in e-commerce. E-commerce businesses try to keep the churn rate at a minimum as it is significantly easier, and less costly, to retain customers, than it is to attract new ones.
These days, the customer journey of less engaged shoppers might have come to the point where they are on the verge of churning. Hence, it is important to predict which customers can be retained, at what cost, and how much they would continue spending at the e-shop (customer lifetime value - CLTV) after being retained.
Reasons for customer churn
There are many reasons why customers churn. When it comes to e-commerce, the reasons listed below are the most common ones:
Limited selection compared to other e-shops
Higher prices compared to other e-shops
Slower or more expensive delivery
Lack of personalization and recommendation of products and services
The customer’s shopping preferences change
Customer base segmentation and churn modelling
When a company finds out they have a problem with customer churn, there are two essential things to analyze – they must conduct customer segmentation and implement effective churn modelling.
Customer segmentation is the practice of dividing the customer base into smaller, more cohesive groups of individuals that are similar in specific ways. These customer groups are then beneficial, for example, in the targeting of marketing campaigns, in identifying potentially profitable customers, or for developing customer loyalty programs. Customer segmentation will effectively uncover which customers have already churned from your e-shop and which customers are on the verge of churning, but you still have a possibility to retain them.
One of the most popular forms of segmentation is called RFM (recency, frequency, monetary), which allows you to divide your customer base by the recency of their last purchase, the frequency of their purchases in a predefined period, and by how much they spent on your e-shop. You can read about an example of successful RFM segmentation in our recent Notino e-shop case study.
Customer segmentation gives you plenty of information about the current state of your customers, which enables you to take many effective actions to retain churning customers. Nevertheless, if you are serious about managing your churn rate, you will need to be able to predict which customers are likely to churn, by when, and with what likelihood. This can be achieved by churn modelling. Churn modelling is carried out via accurate machine learning (ML) models, which process all the customers at once, and find hidden dependencies and patterns in the data. Once such a ML model has been trained, all the past, present, and potential customers can be run through the model. This can give many kinds of results and you should note the following:
If the prediction matches the stable status of the customer, then no action is necessary.
However, if an active customer is likely to flee to another product provider, this will imply that some action to persuade him to stay enrolled in service is needed.
Alternatively, if a customer is currently inactive (meaning that they previously churned) but the prediction is that they will become an active customer, this indicates that they are a good target for a loyalty program in an attempt to recapture the customer’s business.
The predications are also useful to identify the best potential customers and try to win them.
These crucial insights maximize customer preservation and long-term relationship and allow you to precisely target your marketing efforts.
Targeted marketing efforts
When it comes to your existing customer base, customer segmentation and churn modelling will give you plenty of insights to make effective business decisions on. You will be able to make informed decisions and get answers to the following questions:
Which customer segments can be further grown and which need to be retained?
What is the best way to retain the churning customers?
Should we focus our marketing efforts only on the “active” customers given our budget?
How much can we invest in retaining the customers so that our ROI is positive?
What percentage of the churning customers can be retained so that our ROI is positive?
You can use your newly gained information to also attract relevant new customers. If you have access to data about your customers and a list of potential customers, you have an opportunity to focus specifically on those potential new customers who are less likely to churn. This can be achieved by applying algorithms comparing the features and characteristics of your current customers to those of your potential customers. The ones with the most similar characteristics to your current active customers are probably the ones most likely to desire your product or service. These customers will find your offer very valuable and therefore will have the biggest chance to stay with you.
For these reasons, customer segmentation becomes crucial for successful long-term businesses. Each customer segment provides you with distinct features which helps you to understand your customers better and helps you to easily identify both existing and potential customers.
Simplity solution to churn prediction
Simplity has deconstructed the customer churn data requirements, created a new Customer Churn Model, and prepared a solution for churn prediction and management reporting.
Simplity’s solution is based on machine learning, utilizing techniques like decision trees and random forest. One of the benefits of using decision trees, and their variants, is their big variability, which allows them to be used to solve a multitude of business problems. They can also be easily explained, which makes them both easy to understand and easy to use. Using these machine learning techniques allows a business to know their customers better. Combined with an effective marketing campaign it allows businesses to prevent customer dissatisfaction and low service usage.
Data consumed by our method and used for the prediction of customer churn should contain at least: the information about the customer, enrollment type, their usage of your product/services, financial data, and product portfolio structure.
Summary
Customer churn is one of the most important metrics mature e-shops should track because retaining existing customers is cheaper than attracting new ones.
Customer segmentation is the first and essential step in managing your churn rate, as it shows you which customers are currently on the verge of churning. Nevertheless, you can take it one step further by utilizing a machine learning model that can predict those customers who will churn in the future.
If you would like to discuss similar challenges related to churn, get in touch with us.
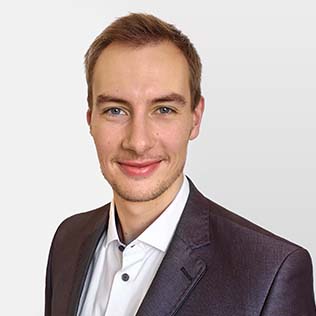